Rust | Getting Started
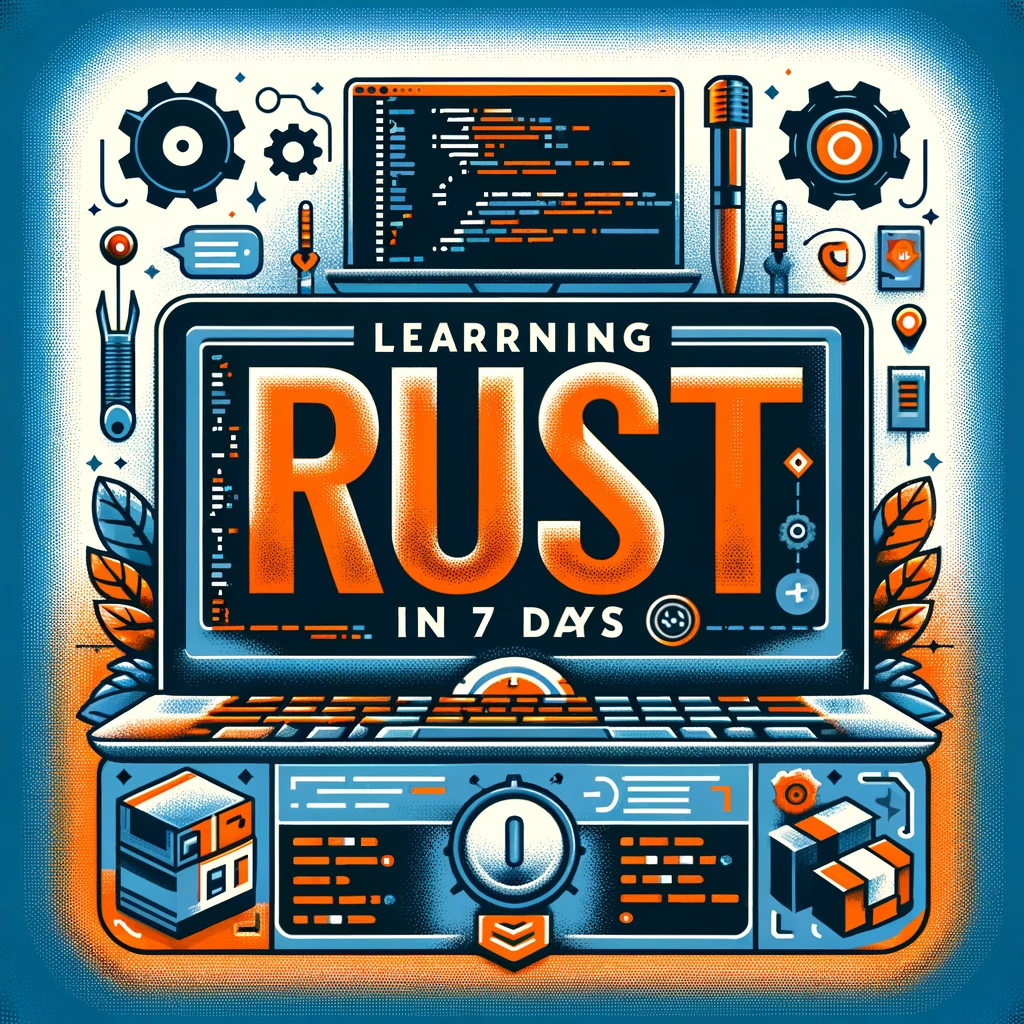
Introduction
From Wikipedia, the free encyclopedia:
Rust is a multi-paradigm programming language focused on performance and safety, especially safe concurrency. Rust is syntactically similar to C++, and provides memory safety without using garbage collection.
Rust was originally designed by Graydon Hoare at Mozilla Research.
It has gained increasing use in industry and is now Microsoft’s language of choice for secure and safety-critical software components.
Rust has been the “most loved programming language” in the Stack Overflow Developer Survey every year since 2016.
Rust could be used in different areas
- Web Assembly
- Embedded Programming
- Command Line Utilities
- Operating System Developmend (see Redox OS)
Read more
- On Stack Overflow : What is Rust and why is it so popular?
- Awesome Lists of Rust Examples:
Installation
Rustup
Download install script and run it
curl --proto '=https' --tlsv1.2 -sSf https://sh.rustup.rs | sh
Modify .bashrc to add Rust path to PATH
source $HOME/.cargo/env
Other way to install Rust on MacOS
brew install rust
Create and run your first App
Create the app
$ cargo new hello_world $ cd hello_world
Show folder structure
$ tree . . ├── Cargo.lock ├── Cargo.toml └── src └── main.rs
1 directory, 3 files
Show main source file
fn main() { println!("Hello, world!"); }
Build your app
$ cargo build Compiling hello_world v0.1.0 (.../hello_world) Finished dev [unoptimized + debuginfo] target(s) in 6.32s
Or build a production ready version
$ cargo build --release<br>Finished release [optimized] target(s) in 0.19s
Run your app
$ cargo run Finished dev [unoptimized + debuginfo] target(s) in 0.04s Running `target/debug/hello_world Hello, world!
Add functionality to your app
Add Dependencies
Let’s add a dependency to our application. You can find all sorts of libraries on crates.io, the package registry for Rust. In Rust, we often refer to packages as “crates.”
In this project, we’ll use a crate called ferris-says
.
In our Cargo.toml
file we’ll add this information (that we got from the crate page):
[dependencies] ferris-says = "0.1"
Modify main source
Now let’s write a small application with our new dependency. In our main.rs
, add the following code:
use ferris_says::say; // from the previous step use std::io::{stdout, BufWriter}; fn main() { let stdout = stdout(); let message = String::from("Hello fellow Rustaceans!"); let width = message.chars().count(); let mut writer = BufWriter::new(stdout.lock()); say(message.as_bytes(), width, &mut writer).unwrap(); }
Run App
$ cargo build Updating crates.io index Downloaded object v0.20.0 Downloaded textwrap v0.11.0 Downloaded adler v0.2.3 Downloaded ansi_term v0.11.0 Downloaded miniz_oxide v0.4.1 Downloaded gimli v0.22.0 Downloaded strsim v0.8.0 Downloaded error-chain v0.10.0 Downloaded vec_map v0.8.2 Downloaded clap v2.33.3 Downloaded smallvec v0.4.5 Downloaded ferris-says v0.1.2 Downloaded backtrace v0.3.50 Downloaded rustc-demangle v0.1.16 Downloaded addr2line v0.13.0 Downloaded 15 crates (1.4 MB) in 1.65s Compiling libc v0.2.76 Compiling bitflags v1.2.1 Compiling gimli v0.22.0 Compiling adler v0.2.3 Compiling rustc-demangle v0.1.16 Compiling unicode-width v0.1.8 Compiling object v0.20.0 Compiling cfg-if v0.1.10 Compiling strsim v0.8.0 Compiling vec_map v0.8.2 Compiling ansi_term v0.11.0 Compiling smallvec v0.4.5 Compiling textwrap v0.11.0 Compiling miniz_oxide v0.4.1 Compiling addr2line v0.13.0 Compiling atty v0.2.14 Compiling backtrace v0.3.50 Compiling clap v2.33.3 Compiling error-chain v0.10.0 Compiling ferris-says v0.1.2 Compiling hello_world v0.1.0 (.../hello_world) Finished dev [unoptimized + debuginfo] target(s) in 14.73s
Run your app
$ cargo run Finished dev [unoptimized + debuginfo] target(s) in 0.14s Running`target/debug/hello_world`
---------------------------- | Hello fellow Rustaceans! | ---------------------------- \ \ _~^~^~_ \) / o o \ (/ '_ - _' / '-----' \
Next steps
Read Getting Started on rust homepage
Explore Learn Rust
Next Readings
- https://blog.rust-lang.org
- https://blog.rust-lang.org/inside-rust/
- https://doc.rust-lang.org/book/
- https://doc.rust-lang.org/rust-by-example/
Readings
- https://github.com/rust-lang/book
- https://github.com/rust-lang/rustlings/
- https://github.com/rust-cli/book
- https://github.com/rust-unofficial/awesome-rust